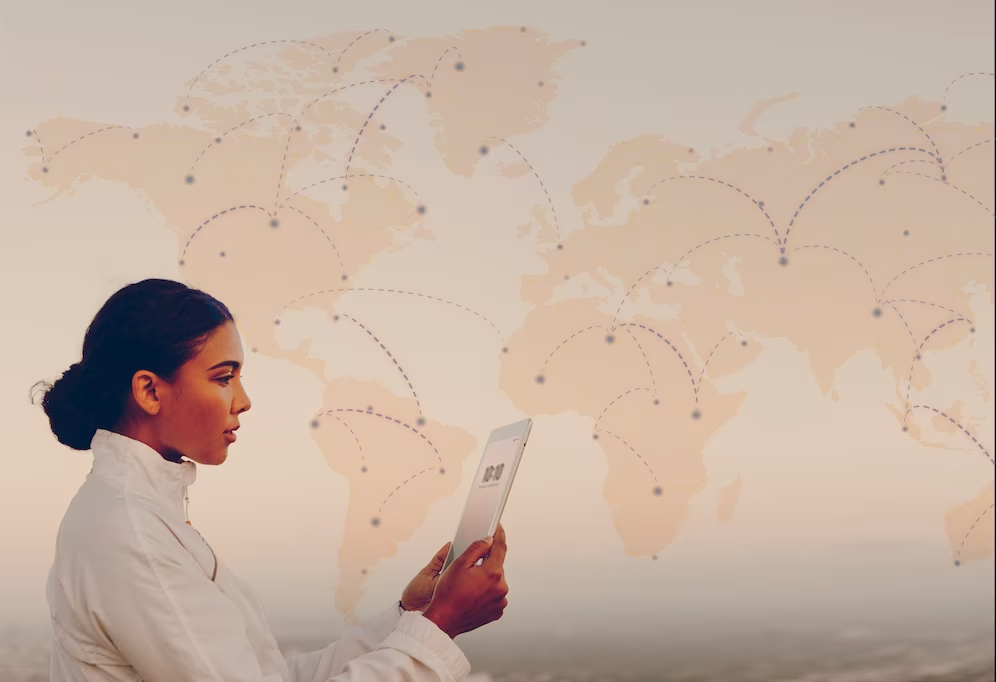
Lead prioritization and analysis is a crucial part of any successful sales and marketing strategy. The need for predictive lead scoring arose from the fact that traditional lead scoring methods, while helpful, often fail to precisely forecast lead conversion.
Predictive lead scoring is a statistical method that utilizes machine learning and predictive modelling to assign a score to a lead that indicates how likely they are to become a customer. This helps sales teams sort leads into groups according to how they think the lead will behave.
The great thing about predictive lead scoring is that it breaks free of the constraints of more conventional methods of lead scoring. Further information about a lead is uncovered by looking beyond traditional demographic and firmographic data into their behaviours, interactions, and even third-party data.
The Mechanism Behind Predictive Lead Scoring
Predictive lead scoring is accomplished via a multi-stage process that includes data gathering, integration, cleaning, model construction, and score assignment. The possibility of a lead becoming a customer can be estimated at each stage. Let’s examine each stage in further depth.
Data Collection
First, a large amount of information about prospects is collected. There is a direct correlation between the data’s quality and quantity and the predictive lead score model’s accuracy. The following pieces of information are usually taken into account:
Data Type | Description |
---|---|
Demographic Data | Age, gender, locality, occupation, level of education, and other personal details. |
Firmographic Data | Specifics about the organization that the lead works for, such as its field, size, location, and yearly income. |
Behavioral Data | Lead activity such as number of email opens, links clicked, materials downloaded, pages seen, time on site, etc. |
Social Media Data | Leads’ interests and degrees of involvement can be gleaned from their social media activity. |
Third-party Data | Use of external data like purchase or credit history to improve lead scoring accuracy. |
Data Integration and Cleaning
After gathering information, the next step is to compile it all in one place using data integration. The leads can now be seen in their entirety thanks to this consolidation. Data cleaning is the process of inspecting a dataset for mistakes, duplicates, and discrepancies and fixing them. This guarantees the quality and accuracy of the data for further study.
Model Building
Model construction is when machine learning methods really shine. A training set and a test set are created from the acquired data. The machine learning algorithm is educated using the training data to learn to recognize patterns and correlates that can foretell lead conversion.
The complexity of the data and the level of precision needed in predictions will determine which method is used. Linear regression, decision trees, and random forests are all examples of popular algorithms. Training and optimization are performed on the chosen method to produce a prediction model.
Score Assignment
After the model is developed and validated on the test set, it is used to give scores to prospects. A score is assigned to each lead that represents how likely it is that they will become a paying customer. A higher score indicates a higher likelihood of a successful sale to the lead. Lead scoring helps businesses focus on the leads most likely to become paying customers, saving time and money in the process.
Collecting a large amount of data on leads, cleaning and integrating that data, developing a predictive model with machine learning techniques, and finally scoring leads based on their likelihood of converting is what constitutes predictive lead scoring. This method equips firms with the knowledge they need to make superior marketing and sales decisions.
The Benefits of Predictive Lead Scoring
Predictive lead scoring provides numerous advantages over traditional lead scoring:
Benefits | Description |
---|---|
Increased Accuracy | Accuracy is much enhanced because more factors, such as complex behavioral patterns, are taken into account in predictive lead scoring. |
Time and Effort Conservation | The time and effort spent on manual lead scoring can be eliminated by switching to an automated system. |
Better Alignment Between Sales and Marketing | Sales and marketing teams can work together more effectively with a single scoring mechanism. |
Scalability | Predictive lead scoring scales well with both data size and company expansion. |
Setting Up Predictive Lead Scoring
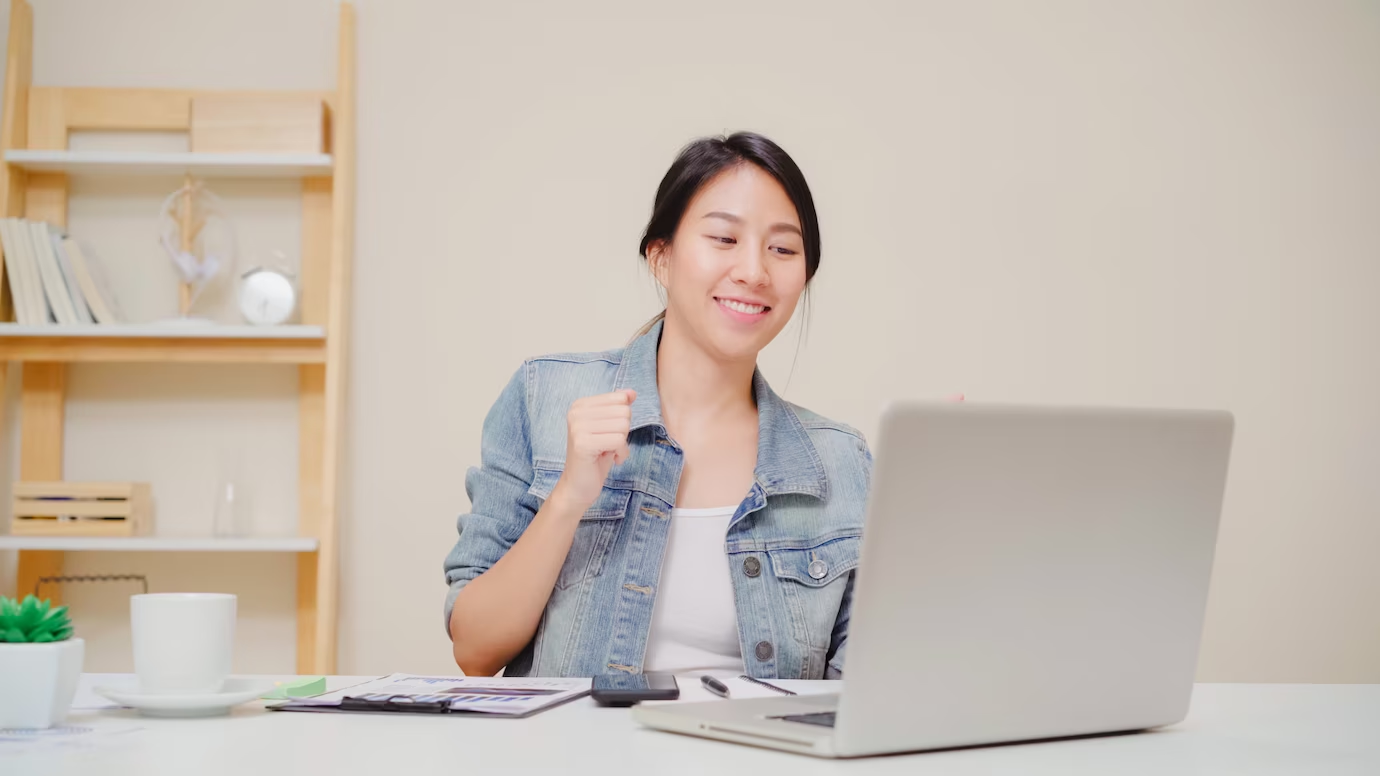
The implementation of predictive lead scoring involves a step-by-step process, which can be broken down into four key stages: goal definition, data collection and integration, selecting a predictive lead scoring tool, and continuous monitoring and refinement.
Goal Definition
The first step in setting up predictive lead scoring is to clearly define your goals. These goals should align with your overall business objectives. For example, you may aim to identify the most qualified leads for your sales team to focus on, increase conversion rates, or optimize marketing campaigns. By establishing clear objectives, you can ensure that your predictive lead scoring efforts are targeted and effective.
Data Collection and Integration
Accumulating relevant data from various sources is crucial for creating a robust dataset for analysis. This data can include both internal and external sources, such as customer demographics, website interactions, purchase history, social media data, and more. The more comprehensive and accurate your data, the better insights you can generate from your predictive lead scoring model.
To collect and integrate data effectively, consider the following steps:
- Identify the data sources: Determine which sources contain the most valuable information for lead scoring. This could include your CRM system, marketing automation platform, website analytics, or external data providers;
- Extract and transform data: Extract the necessary data from each source and transform it into a consistent format for analysis. This may involve cleaning and organizing the data to ensure its quality and reliability;
- Integrate the data: Combine the data from different sources into a single dataset for analysis. This integration can be performed using data integration tools or by developing custom data pipelines.
Selecting a Predictive Lead Scoring Tool
Choosing the right predictive lead scoring tool is crucial for the success of your implementation. Consider the following factors when selecting a tool:
- Integration capabilities: Ensure that the tool can seamlessly integrate with your existing systems, such as your CRM, marketing automation, or customer support platforms. This integration will enable real-time data updates and efficient lead management;
- Customizability: Look for a tool that allows you to customize the lead scoring model according to your specific business needs and objectives. This flexibility is essential as it enables you to adapt the model as your business evolves;
- Model performance and accuracy: Evaluate the tool’s track record in terms of model performance and accuracy. Look for customer reviews, case studies, or benchmark reports to assess the tool’s effectiveness in predicting lead quality;
- Scalability: Consider the scalability of the tool to accommodate growing data volumes and increasing business demands. A scalable tool can handle larger datasets and ensure consistent performance as your lead generation efforts expand.
Continuous Monitoring and Refinement
Once your predictive lead scoring model is up and running, it’s crucial to monitor its performance regularly. This monitoring helps identify any issues or areas for improvement, ensuring that your model remains accurate and effective over time. Consider the following practices for continuous monitoring and refinement:
- Establish performance metrics: Define key performance metrics to measure the effectiveness of your lead scoring model. This could include conversion rates, sales revenue, or customer lifetime value. Regularly analyze these metrics to assess the impact of your model on your business outcomes;
- Feedback loop: Establish a feedback loop with your sales and marketing teams to gather insights on the quality of leads generated by the scoring model. This feedback can help you identify any discrepancies or areas for improvement and refine your model accordingly;
- Model updates: As your business evolves and new data becomes available, update your predictive lead scoring model to reflect these changes. This ensures that your model remains relevant and accurate, accommodating shifts in customer behavior or market dynamics.
By following these steps and implementing a well-defined predictive lead scoring process, you can optimize your lead generation efforts, improve sales and marketing alignment, and drive better business outcomes.
Common Misunderstandings Around Predictive Lead Scoring
Predictive lead scoring, while revolutionary, is not without its misconceptions:
- Exclusivity to Large Businesses: Predictive lead scoring is not exclusive to large enterprises. Businesses of any size can harness its potential;
- Irrelevance for B2C: Although often associated with B2B sales, predictive lead scoring can be equally valuable in a B2C context;
- Replaces Sales Teams: While it can automate the process of scoring leads, predictive lead scoring doesn’t eliminate the need for sales teams. It merely aids them in their tasks.
Conclusion
Predictive lead scoring is an effective method for organizing leads and directing sales and marketing resources. It promises improved business outcomes by utilizing machine learning and big data analytics to deliver a more accurate, efficient, and scalable solution for lead scoring.
FAQ
Predictive lead scoring enhances sales efficiency by providing a data-driven, objective basis for prioritizing leads. This helps sales teams to focus their efforts on the most promising leads, increasing the likelihood of conversions and boosting overall sales performance.
Yes, it’s necessary to continuously refine and update the model. Market conditions, customer behaviors, and business goals can evolve, so your model must adapt to remain accurate and effective.
Machine learning plays a crucial role in predictive lead scoring. It allows the system to learn from the data, identify patterns and correlations, and predict future outcomes.
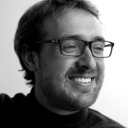
Mark is an experienced internet entrepreneur and marketer whose career in lead generation started relatively recently but has quickly gained momentum. He/she has in-depth knowledge of internet marketing and customer acquisition strategies.